Home > News & Events >
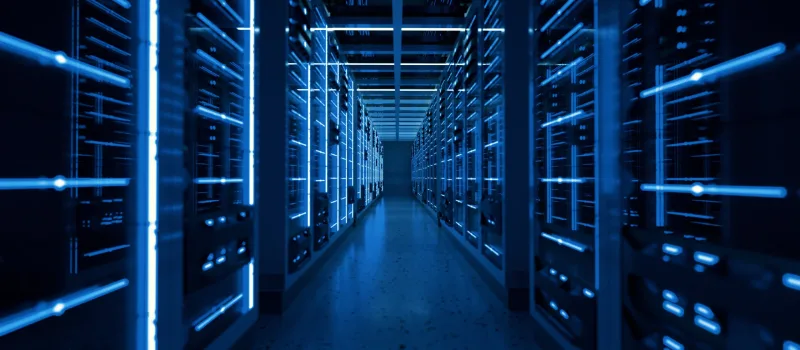
CoreStation AI Magic!
24 September 2024
How to fit 30 GPU cards in a 0.5U remote workstation for AI Workloads
Before I fully explain the title of this blog and put some context around what it actually means for an organization, I thought I would start by setting the scene around what I mean when I talk about AI workloads, what they are, how quickly the market it is growing, and then the typical challenges an organization can face when adopting an AI strategy.
Firstly, why is the whole AI thing important in the first place and how fast is it growing?
To answer the growth part first, the AI market is growing at an annual rate of around 19% and accounted for $638.23 billion in 2024 and is projected to reach $3,680.47 billion by 2034 as shown in the graph based on market size research from Precedence Research.
Why is AI growing?
As is typical with any information technology solution, the technology is getting better and faster with the ability to squeeze even more processing power onto ever decreasing silicon sizes (remember Moore’s Law?), which typically also drives down the cost of ownership. Therefore, making the technology more available.
It is also down to the sheer size and amount of data that is out there that needs harnessing and processing to make use of it. Which brings me nicely onto the types of workloads that AI embraces.
I’ve already touched on data processing where data needs to be collected, analyzed and interpreted. These can range from data processing and machine learning model training to real-time inference and decision-making.
Maybe for data scientists dealing with vast amounts of scientific data for research, or quantitative finance where you need to predict the way markets are moving. Not to mention media and entertainment for tracking preferences and suggesting personalized content.
What are the Challenges when Deploying AI?
First and foremost, to run AI-centric workloads you will need multiple GPUs in your workstations. And therein lies the first challenge. A typical workstation machine may be able to accommodate one or two GPU cards at best, but typically no more than that. Certainly not enough to deliver the performance required for AI workloads. So, scalability is the issue here.
The answer then surely is to have more workstations and therefore more GPUs. Well, not really as the GPUs are wedded to the individual workstations and cannot be shared to provide the total amount of resources required. In any case, it wouldn’t be very economical to buy that many workstations or GPUs for that matter, as likely the full performance won’t be required all of the time. Don’t forget GPUs are not the cheapest component. The challenge here is the flexibility, in that it isn’t very flexible at all.
The question is, how can these challenges be solved?
How CoreStation Solves the Challenges
With the Amulet Hotkey CoreStation for AI solutions, organizations can solve both the scalability and flexibility issues that can hold them back from adopting AI.
If we start by addressing the flexibility challenge.
By adopting a datacentre model, CoreStation for AI abstracts (or removes) the local GPU cards from the workstations and creates a centralized pool of GPU resources by using an external chassis (each chassis capable of taking up to ten GPU cards) and a PCIe fabric, or network if you like) to connect workstations to GPU’s. Think along the lines of a storage area network but for GPU cards now instead of disks.
Next, as we are in the datacentre now, we can address the scalability. Workstations are deployed using density optimized rack mounted enterprise-class hardware. For example, with the CoreStation for AI CX solutions, you can deploy four workstation nodes into just 2U of rack space. That means each workstation is just 0.5U in size. As workstations are now remote, users connect directly to their workstation from a client on their desk. Being in the datacenter now means you can easily add additional workstations as you grow.
Let’s now bring the workstation and GPUs together. By having an on-demand pool of GPU resource, via the management software, you can now dynamically allocate GPUs to the workstations. If I take you back to the title of this blog, this is where the ‘magic’ happens.
If you have three fully populated chassis, each with ten GPU cards (30 GPUs in total), you could allocate all 30 GPUs to just one of those workstations, and, abracadabra, you have a 0.5U workstation configured with 30 GPU cards. All simply done with just the click of a mouse!
What Other Benefits are There?
The CoreStation for AI solution easily addresses the flexibility and scalability questions; however, it delivers benefits far beyond the physical hardware capabilities.
Secure by design
As I’ve said before, with AI comes vast amounts of data and all the risks associated with storing and managing data and legislation that comes with it. With CoreStation for AI being a datacenter solution then the data stays within the confines of the datacenter. It doesn’t leave and so by connecting remotely, no data is left on local devices.
Improved user experience
I once worked on a trading floor project and the multiple workstations on each desk, coupled with graphics cards that get very hot and are very noisy when the fans arere all running doesn’t make for a great working environment. By moving these heat generating workstations, not to mention the increased power requirements, all aid in creating a better desktop environment.
Not only that, but users can also work pretty much from anywhere without any compromise on performance when it comes to running AI workloads.
Summary
To summarize, the Amulet Hotkey CoreStation for AI solution enables an organization to embrace and adopt AI-centric workloads empowering employees by delivering the performance they require, when they require it, and from wherever they are working from.